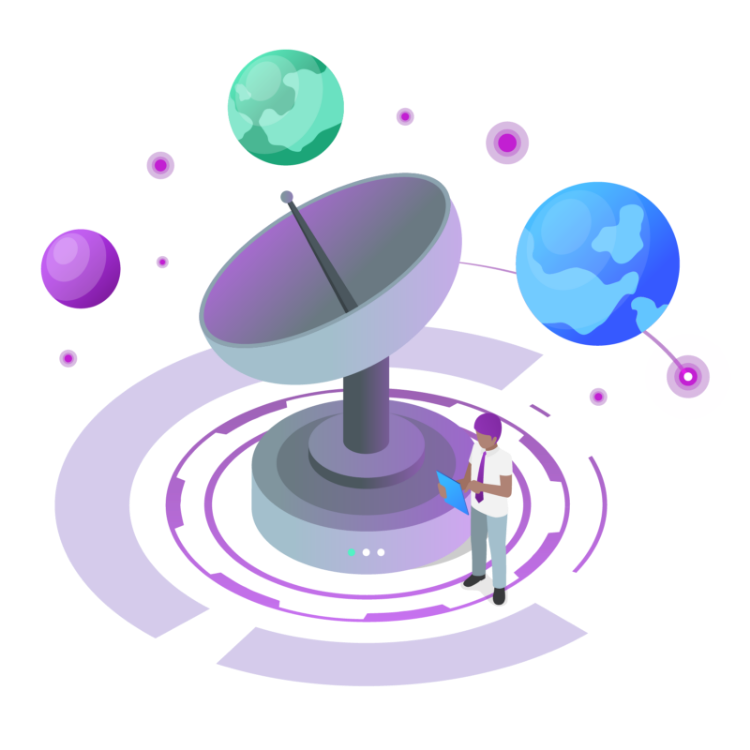
The ask
A European Space Agency research group uses a technology called Spectral Energy Distribution (SED) to analyze proto-solar systems. They have a very well-defined and complex mathematical system in place that provides accurate results regarding the existence of the crucial elements needed for life. Each analysis takes 52 hours to compute, and there are hundreds of parameters that must be tested. The client was looking for a way to improve the performance of this task by enhancing their existing SED technology.


KEY OBJECTIVE: REDUCE COMPUTING TIME OF ANALYSIS BY ENHANCING A COMPLEX MATHEMATICAL SYSTEM FOR A EUROPEAN SPACE AGENCY, WITH DATA SCIENCE.
What we did
To achieve this objective, we identified Deep Learning as a potential approach to making use of the client’s high volume of historical data. This is due to Deep Learning’s ability to approximately predict results given a specific input, and accurately mimic hyper-complex mathematical systems – such as those based on physics and chemistry.
Because of its diverse range of applications, Deep Learning can have a significant impact on processes when used in industries such as pharmaceuticals, aeronautics, energy, and many more.
The process

We started by collating all relevant data, including inputs and results of past experiments. This allowed us to better understand what had happened historically when these were run.
Next, we trained several Deep Learning models on this collated data. Once the models were well trained, they could then be applied to new SED datasets, and their performance and accuracy could be measured.
Due to the volume of data, and the complexity of the models we were testing, we used the Intelygenz technical environment to define the best results prior to transferring to the client. We then embedded the solution into the client’s workflow for astrophysicists to start using.
The result
Using the client’s SED system and previous methodology, it took an average of 52 hours to run an experiment. But with the new Deep Learning solution in place, the same experiment takes only 5 hours, while still providing an equally accurate result. This reduced total computing time by 90.4%.
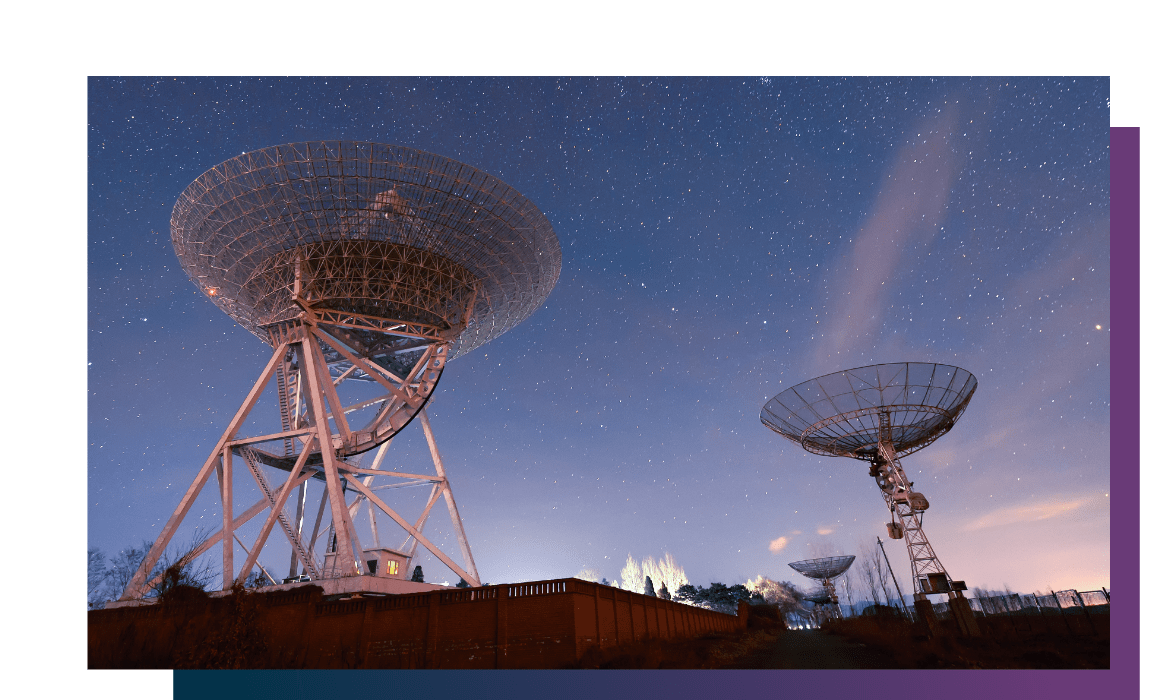

EXPERIMENT RUN TIMES REDUCED BY 90.4% WHILE RETAINING RESULT ACCURACY.
Following testing in real scenarios, we identified that the same Deep Learning model could be used to also simulate algorithms and propose
experiment parameters for use with the existing SED technology.
The final solution helps Astrophysicists speed up their research and optimize their complex systems, enabling them to make informed decisions in less time.